Customer behavior prediction
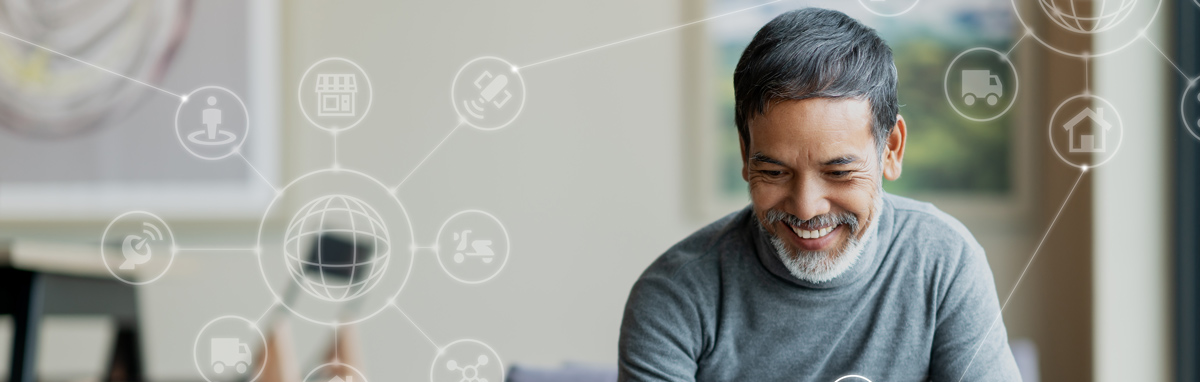
THE COMPANY
Our client is a United States retail chain business with a seventy year of history, over 7,000 employees, 13 million customers, and more than 480 branches in nine states of the western United States.
THE CHALLENGE
This company had to make a complex decision to close and reconfigure some of its branches, as part of a reorganization process during the Covid-19 pandemic. Our client needed to be able to count on reliable information that would enable the making of good decisions fast.
To do this it was necessary to predict the following:
- The number of clients that would be lost due to the closings.
- The impact of the closings on global sales.
- The most efficient measures for mitigating the loss of clients as much as possible.
- The customers they would most need to retain.
THE SOLUTION
By using machine learning, artificial intelligence, and data lake techniques, we were able to gather and cross data on user behavior in order to develop algorithms that predicted, with some precision, not only the percentage of customers that would accept a move to another branch, but also which branch they would migrate to, as well as those customers who would not move and who would look to the competition for alternatives.
In addition, we implemented graphic visualization technology with maps to simulate how customers would react to a given campaign or to the absence of any action. This made it possible to carry out segmented and focused campaigns in accordance with knowledge of the customers for the purpose of directing them to a branch where they could have the same type of experience they were used to.
Not all customers are the same, so we used algorithms to determine what offers might interest our client´s customers based on their history of product purchases, the frequency of these purchases, the average sales receipt, how the customers reacted to promotions, and the channels and times of preferred purchases. Likewise, not all customers generate the same revenues, which is why we incorporated discounts that were differentiated according to brand values.
BENEFITS
- Through targeted actions, 34% of clients were retained.
- A new company asset in the form of a data-driven model for decision making.
- An improved customer experience thanks to an increased capacity to personalize offers.
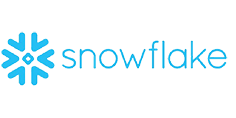
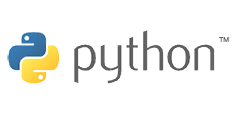
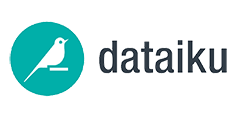
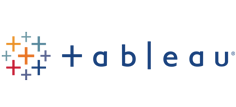